Socio-technical approach for the creation of a decentralized data architecture: Data Mesh
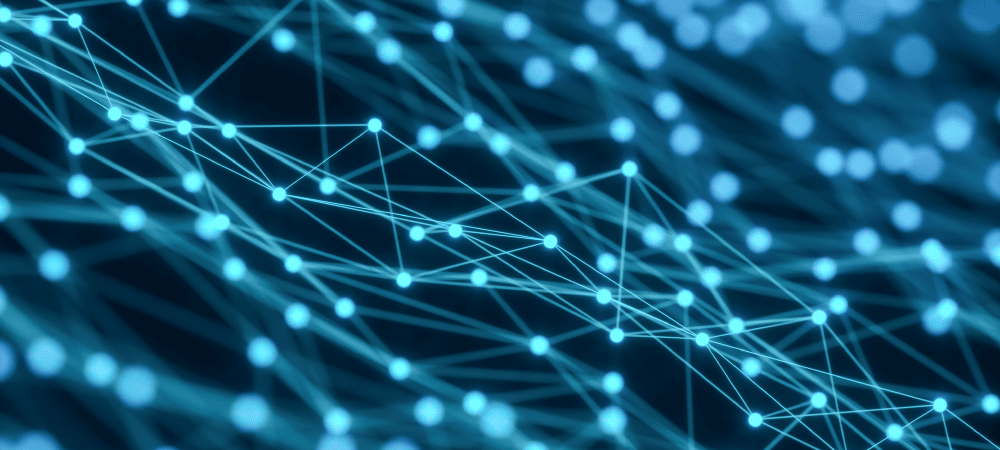
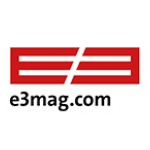
New data sources and diverse data formats make data management a complex challenge for companies. Various isolated solutions, long development times and a vague value contribution are frequently lamented problems. IT managers and those responsible for SAP must therefore ask themselves the question: How can data succeed in delivering the desired value contribution?
The solution is an efficient, effective and future-proof data value chain. The data value chain is an ideal-typical model that shows the path of data in the company from the creation of raw data to insightful business decisions. It consists of four phases:
In the first phase, data creation, raw data is generated manually or automatically, for example digitized receipts, digital transactions or IoT device data. This data is then processed operationally in the second phase and used in the corresponding work steps. One example is resource or capacity planning for production machines or stock levels in SAP ERP solutions.
After the operational processing of the raw data, the third phase involves analytical processing. Here, state-of-the-art tools and methods translate data into further insights that can be used directly.
In an SAP environment, for example, this can look like this: Various data sources, which can also be solutions from other cloud providers, are connected to the SAP database, for example with the SAP Hana Cloud. SAP Data-sphere can be used as a warehouse solution, the SAP Data Intelligence Cloud for orchestrating and pipelining the data. The SAP Analytics Cloud can then be used to carry out analyses and implement advanced planning scenarios. The data processed in this way is in turn the basis for the fourth phase, in which it is used for data-based decision support or even to make decisions autonomously. This phase also includes strategically aligning the company on the basis of refined operational and analytical data.
Data value chain
In order to organize the data value chain in such a way that data actually delivers business value, major transformation efforts are generally required. A distinction can be made between soft or socio-cultural aspects and hard or technical aspects.
If companies focus on soft aspects, they aim to offer employees skills or framework conditions in which they have rights and obligations as a data society. Specifically, this involves data literacy programs that train employees in handling data. Or establishing a culture of innovation for the digital world. If, on the other hand, companies focus on the hard aspects of transformation, the focus is on building a standardized platform that enables the seamless storage, processing and retrieval of data.
However, both approaches in isolation neglect the synergy effects that can arise when soft and hard aspects are combined. The data mesh is such a combined approach that allows different organizational units or domains to produce, own, use and share data products. At the same time, the gaps in technical integration and cultural acceptance are bridged.
How does Data Mesh work?
At the heart of the data mesh concept are, on the one hand, decentrally organized domains that are oriented towards the business context of the data assigned to them and, on the other hand, data products whose data records provide a benefit. This is supported by a federated governance that can be executed as automatically as possible and the existence of self-service on the basis of a harmonized technological platform.
By combining these four principles, the data mesh concept cleverly links decentralized and centralized aspects of data management. In this way, responsibility for the creation, publication and maintenance of data products is transferred to the domains, i.e. the functional units of a company. This is because this is where both the need and the content expertise are to be found.
For example, financial planning for a tactical period of six months, which is made available as a data product, can be assigned to the domain for financial data. In addition, the technical teams are reorganized into platform and domain teams. This makes it possible - without the bottleneck of a central IT/development department - to develop decentralized data products according to centrally coordinated guidelines. In this way, the balancing act between the autonomy of the domains and the divisibility or reusability of the data products is achieved.
The introduction of a data mesh requires extensive changes in various areas. Practical experience shows that the following factors are crucial for successful implementation of the data mesh approach: vision and strategy; rights and responsibilities; readiness for change; governance and standards; technology and infrastructure; skills and capabilities; and communication and collaboration. Companies should see the data mesh as a journey, during the course of which the concept can be continuously developed individually and on the basis of initial milestones. Accordingly, the factors described should not be regarded as exhaustive, but they do provide a good insight into aspects that are particularly critical to success.
