AI in Retail
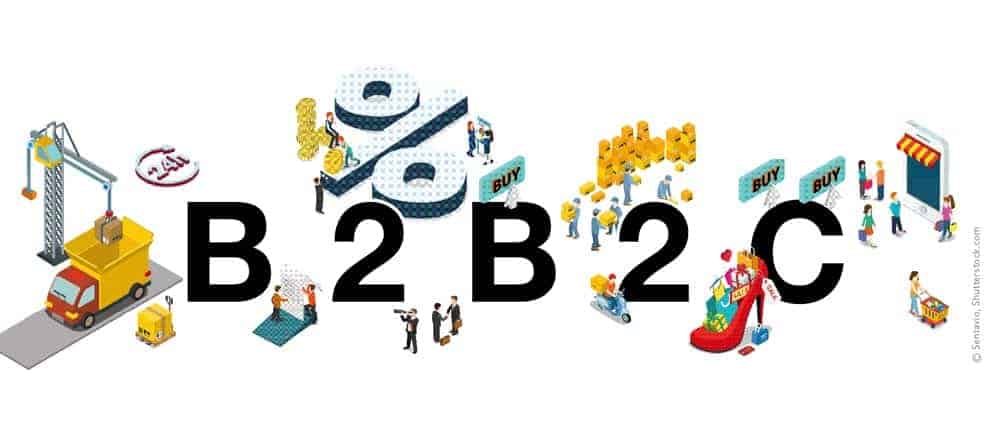
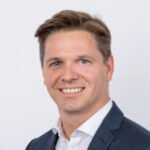
The retail sector has long been under pressure due to customers' increased price sensitivity and the general pressure on margins. To counteract this, digitization initiatives aim to optimize processes such as purchasing and replenishment planning, stimulate additional sales through personalized sales recommendations and make prices and product ranges more efficient. The biggest challenge is the immense amount of article and customer data. Although big data opens up interesting application scenarios for artificial intelligence, such as cashierless stores, the high data quality required for this is particularly crucial for invisible processes that run in the background.
The challenge of data quality
A key difficulty for retailers is harmonizing sales across different sales channels such as brick-and-mortar stores, online marketplaces and stores or catalog sales. The amount of product information is constantly growing, while at the same time the product range is subject to constant change. Retailers therefore have to record and mutate large quantities of new product data records with up to hundreds of attributes each day. In addition, the inclusion of structured and unstructured data from product images and videos is becoming increasingly important due to growing e-commerce. This data maintenance is time-consuming and error-prone, as it is based on the incoming product information and the numerous rules derived from it. Incorrect data quality can have devastating consequences such as incorrect deliveries and different prices for identical products. On top of this, there is the threat of declining customer satisfaction, which spreads quickly in the age of social media.
However, rules for master data maintenance can cause difficulties for established ERP systems such as SAP for Retail. Retailers therefore often resort to in-house developments to expand their ERP. This is associated with high maintenance costs, reduced system flexibility and inadequate functionalities. Standardized add-ons that can be integrated into the ERP without modification can provide a remedy.
Standardized AI extensions
Machine learning, a sub-discipline of artificial intelligence, makes it possible to create and adapt test rules flexibly and without involving the IT department. Algorithms identify plausibility rules, carry out error corrections autonomously or automatically make suggestions for corrections to the user. The information gained from this flows back into the system as training data, allowing the system to gradually learn the correct classification itself.
AI at the beginning of a development
Such partial automation using artificial intelligence reduces error rates and process costs and ultimately enables optimized replenishment or action planning as well as store-specific assortment planning. Retailers can now also work with their suppliers to access weather, event or geodata in order to improve the planning and availability of products and pursue zero-waste projects. The quality of article data is therefore particularly important for the efficiency of merchandise management processes. Continuous further development and the integration of AI solutions promise to efficiently overcome the hurdles that still exist today and significantly increase system performance in the future. Sophisticated master data maintenance paves the way for future-oriented AI applications such as just-walk-out stores, intelligent shopping baskets, digital mirrors and other smart applications that could revolutionize the retail industry.