The final technologies to solve all IT security problems
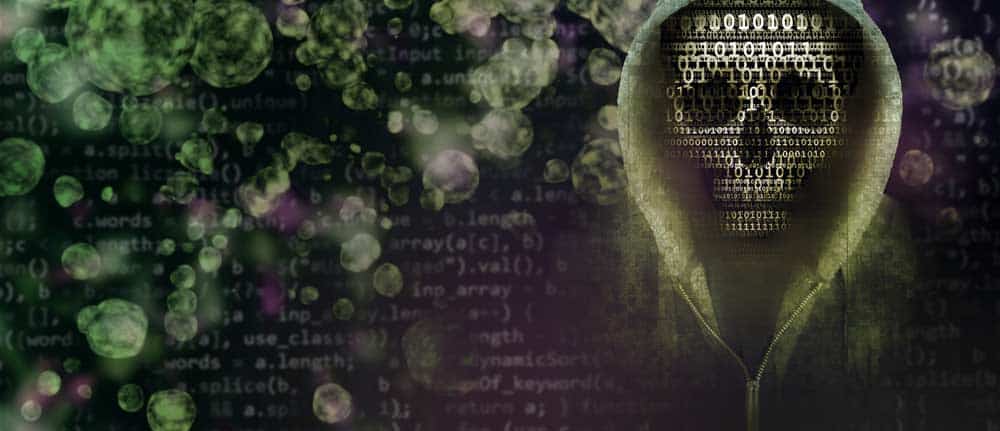
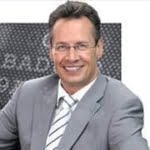
But just as theory differs from practice, one must distinguish between technology and its implementation.
A technology that is recognized as "safe" according to the current state of research may nevertheless be unsafe in a concrete implementation.
This is what happens with public key infrastructures (PKI). The theory behind it - i.e. symmetrical and asymmetric encryption, digital Signatures and certificates - are established and proven technologies.
Nevertheless, the implementation of PKI has weak points. In this specific case, two "certificate authorities", namely Startcom and Wosign, issued false or at least questionable certificates.
The technology behind it worked flawlessly.
Nevertheless, the incorrect or improper use or implementation created a security problem.
So if you limit yourself to just the technology view, everything was flawless - yet there were gaps in the implementation and thus security problems.
Insecure certificates despite secure cryptography. Now cryptographic algorithms and PKI, at least in our IT age, very old. So to speak from the stone age of computer science...
So the current technology buzzword is "Machine Learning"especially in the IT security sector. If you believe some marketing statements, this makes machine learning all other technologies obsolete.
But also ML is "only" a technology. And strictly speaking, it's not even new: Many basic algorithms and procedures have been known for decades.
Even though ML as a technology has a lot of potential, especially in detecting new unknown threats, it is well worth looking at the implementation.
One of the most important factors in implementing ML is training. This includes the amount and quality of training data as well as the training method.
The quality of an ML implementation thus depends directly on the quality - and to some extent the quantity - of the training data. It is therefore not enough to have a good command of the pure technology ML.
Rather, external factors, in this case the training data, also play a - if not "the" - decisive role. To put it casually: "Garbage in, garbage out."
Machine Learning vs. Training
In addition to the "technology" dimension, there is suddenly another dimension to consider when evaluating ML implementations: the "training set."
This is an example of how a technology must always be evaluated in the context of its implementation.
Another aspect that implementation can throw a spanner in the works is "false positives". That is, legitimate content that is incorrectly classified as undesirable.
Many ML algorithms historically suffer from this problem. They may very efficiently detect new threats that other technologies do not, but at the same time report a lot of harmless content as dangerous.
In the context of an optimization, therefore, further noise reduction measures are often used.
The influence of new technologies is indispensable for IT security. If only to be able to meet the creativity of cybercriminals on an equal footing.
However, technology affiliation does not lead to the desired results. The implementation and its context must always be considered.
If this is not done, there is always the danger of creating the impression of a "perfect" technology. A theory that is all too often disenchanted by practical implementation.