APS autopilot and resilience
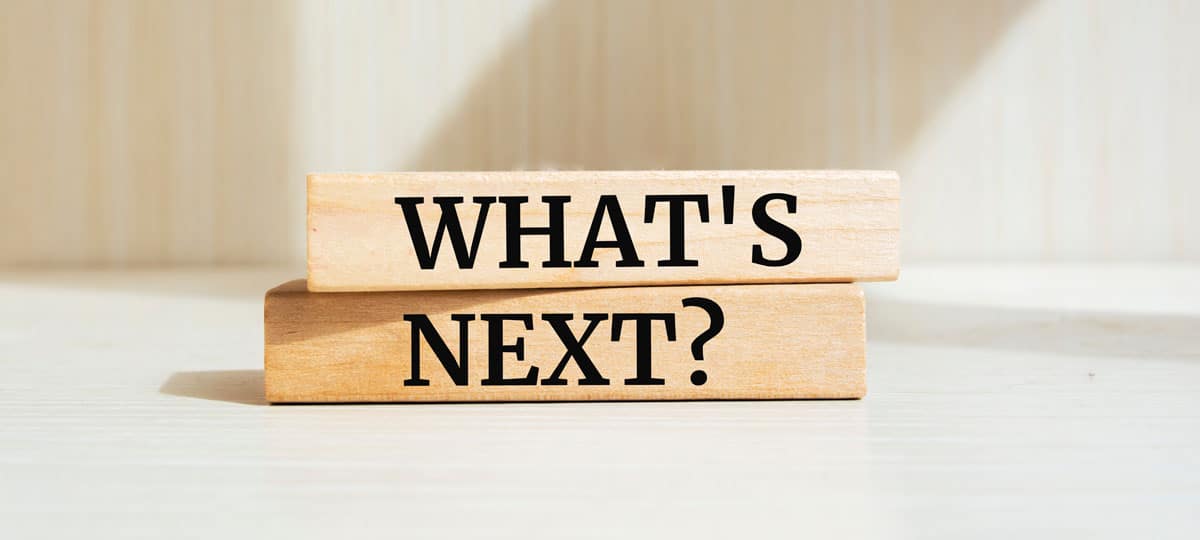
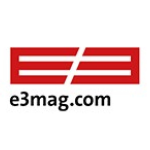
Industrial detailed planning is in the midst of tremendous change
A new generation of APS (Advanced Planning and Scheduling) solutions is ready to go, based on AI and data science for responsiveness and thus significantly higher output and shorter lead times. In this interview, Daniel Poodratchi, Chief Product Officer at APS specialist Anacision, talks about what is already possible in this area in the SAP environment today and what basic conditions must be met.
AI in production, where and how is the technology used?
Daniel Poodratchi, Anacision: On the one hand, there is predictive maintenance using AI-based data analysis - an ongoing topic in recent years that has not met expectations because the cost-benefit ratio is often not given. The individual adaptation of the solutions to the respective type of operating equipment is very time-consuming with most approaches. Especially for plant operators who use very heterogeneous equipment, there is therefore usually no use case. Automatic optical inspection, on the other hand, works very well in some areas.
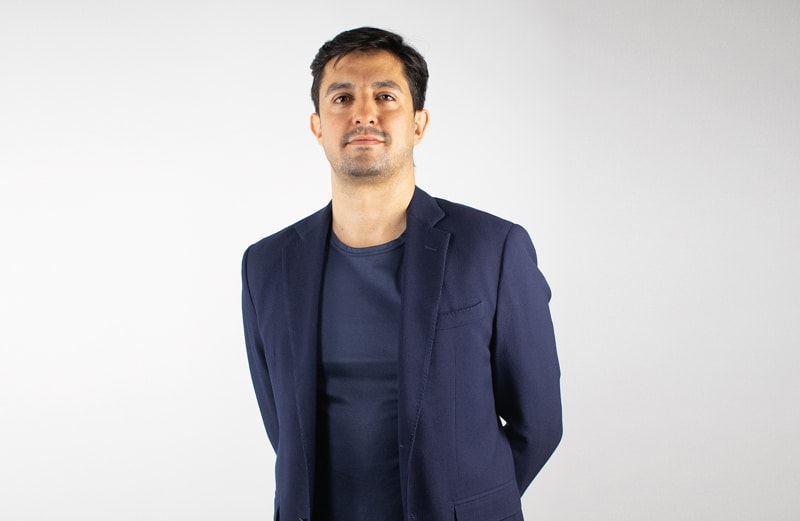
"In this way, quality inspection can be raised to a new level without human intervention.“
Daniel Poodratchi,
Chief Product Officer, Anacision
Their potential has once again increased considerably in the area of "end-of-line testing," for example. This allows quality testing to be taken to a new level without human intervention. And last but not least, there is the area of detailed planning. The special thing here is that if you turn the right screws, this often produces a considerable optimization effect. After all, it's not just about one machine or line, but about the detailed planning of an entire production site.
In which direction is the topic developing from your point of view?
Poodratchi: First of all, be aware of the direction in which our world has developed. There is more complexity than ever before and more and more short-term, impossible-to-plan events challenge planners in their daily tasks. Constantly changing orders, staff shortages and the declining level of education among plant employees make the task even more difficult. Against this background, it is clear that companies need to become much more flexible in their production to meet these challenges. And for this flexibility, high-performance detailed planning is indispensable, otherwise the actual potential of production will remain unused. New technological approaches in APS solutions open up enormous possibilities here. At the same time, the project effort for implementation is significantly lower compared to classic APS solutions.
Which technological approaches open up new potentials in detailed production planning?
Poodratchi: Three topics play a role here: First, new algorithms from the AI environment in conjunction with the availability of cloud computing. These enable highly efficient plans for individual production structures to be calculated within seconds.
Secondly, networking along the supply chain, see SAP IBP, Integrated Business Planning, and in production, for example via IoT, MDE and BDE, is increasing all the time. Taking this data into account in detailed planning opens up unprecedented opportunities in terms of responsiveness, automation and optimization.
Thirdly, the increased use of AI helps to perfect detailed planning in a self-learning manner. Incidentally, we also have a clear target picture in this context: The entire solution ultimately functions like a navigation system in a car. In other words, it shows the ideal route to the production destination, predicts the arrival time (delivery date), reacts directly to deviating events, and then calculates a new ideal route in a matter of seconds.
Which functions distinguish your solution and where does its operational added value lie?
Poodratchi: Ultimately, APS is always about a holistic view of production processes in order to be able to realize the most effective optimization potential. We are therefore talking about a very strong lever for key performance indicators such as throughput, on-time delivery or lead time. These are areas where the optimizations - and thus the success of our solution - can be measured very precisely.
Specifically, what does the customer get?
Poodratchi: Customers do not get a black box. We look at the historical production data for a period in advance and use it as the basis for creating an optimal plan. Such a test plan then makes the achievable KPI optimizations as well as savings potentials very concretely visible. In addition, companies can test the feasibility of the plans. In short, it shows whether the solution is worthwhile at all. To make the dimensions clear: In practice, for example, we succeeded in increasing the output of a plastics manufacturer by 17 percent and the capacity utilization of a sensor manufacturer by 10 percent.
Thank you for the interview.
