Big Data = Big Business?
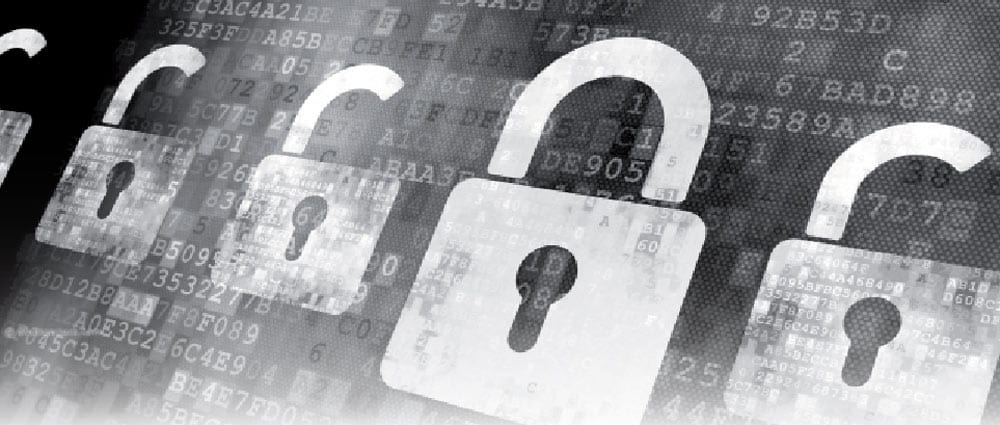
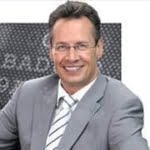
At the recently concluded Black Hat conference, the new magic word seemed to be "Artificial Intelligence", artificial intelligence. The goal is no longer "only" to obtain usable data from the available data sources by means of an intelligent algorithm - but also the automated (finding) of the appropriate algorithm.
The background to this is certainly also the observation that the IT side of Big Data can be scaled, while the necessary human creativity and experience is much more difficult.
The Cyber Grand Challenge, whose organizer, DARPA, manages research projects under the U.S. Department of Defense, shows where the journey can lead.
Put simply, this competition is intended to create autonomous systems that can detect and close security gaps. What at first sounds like the "next Holy Grail" of defending against vulnerability attacks becomes much more explosive when you realize that the systems are competing against each other in what is known as a capture-the-flag scenario - and are not only trying to automatically find and close vulnerabilities in their own systems, but also to find and exploit them in the others.
Many technologies are not clearly good or evil, the decisive factors are the application scenario and the intention!
But even despite the connection to the U.S. Department of Defense, one should be careful with a general suspicion. After all, public DARPA-funded research projects gave rise to many technologies that we perceive as "good" today as a matter of course.
The most prominent example is the Internet.
Even if the results of the Cyber Grand Challenge were impressive, we are still a long way from an autonomous system that matches the capabilities of a human being "in production". The dual (mis)use scenarios for big data technologies are much further along.
Phishing, for example: Everyone is probably familiar with the fake cell phone bills or package notifications designed to lure unwary users to phishing sites.
In the corporate environment, they are even worse than so-called spear phishing emails - i.e., emails that are intended to lure a person or group to websites. Mostly as a precursor to a targeted attack.
Against this background, it is hardly surprising that many solutions for "big data-based threat detection" are dedicated to the detection of phishing. The findings from big data analyses are used, true to the motto "put the good ones in the pot, the bad ones in the jar".
However, the same data can also be used to draw conclusions about which persons/targets are particularly promising, which phishing content is clicked on particularly often and, as a final consequence, is not detected by security solutions!
This is exactly the scenario outlined by SNAP_R, a tool presented at Black Hat: It automatically generates a "hit list" of worthwhile targets from public Twitter data and a list of targets - and, based on content in their timelines, automatically tweets with links.
These are proven to be clicked more often than comparable mass phishing tweets.
Although SNAP_R was developed as an automated-spear phishing tool for penetration testers, there are no limits to its (malicious) use. Regardless of the specific tool, this is also evident here:
A technology is often neither clearly "good" nor "evil."
Rather, history teaches us that it depends on the context and the person using it. In the context of IT security, there are technologies (current and future) that on the one hand can help to better detect and defend against attacks, but on the other hand can be used to optimize precisely these attacks against defensive measures.
For all our love of technology, we should not wait for the "grail of IT security" - but use available technologies sensibly after a risk assessment.