Can AI also do ERP?
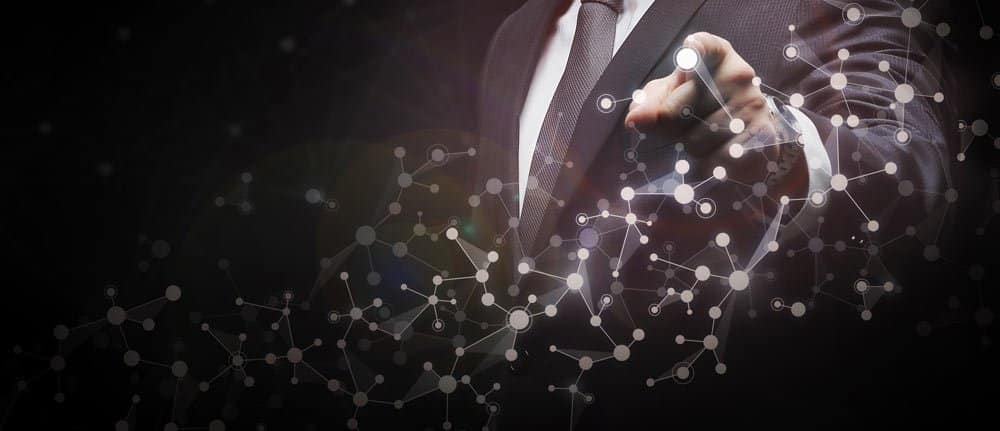
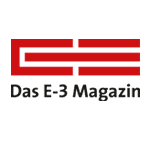
The recent boom in AI, particularly in the area of language processing, has been fueled by advances in deep learning techniques and the availability of big data. This has enabled AI models such as ChatGPT to understand and produce natural language text with remarkable accuracy and fluency.
And that's exactly the kind of text you've just read. Impressive, isn't it? But how do we get from generated texts and images to operational business processes and our beloved ERP software?
First, let's take a quick look at the track record of intelligent approaches in enterprise use. You have certainly used a support chat at some point. Their "intelligence" is usually characterized by the fact that exactly when real intelligence was required, it was handed over to a real employee. Such rule-based chatbots, which are mainly used in the service and support area, can effectively process simple routine business transactions. However, their function is mostly limited by the "knowledge" they were previously taught by developers, and thus they do not fall under a narrower definition of AI in this form.
In addition, many companies are already using tools that enable automated processes through machine learning, such as document classification in the inbox, spam detection for e-mails, or fraud detection in the financials environment. In the next step, however, we are talking about an AI that can not only tell a user the preliminary company profit for Q4, but can also explain why this profit deviates significantly from Q3.
Every AI must first be trained with knowledge in order to deliver meaningful results. But what is knowledge in a corporate context? Knowledge about processes is predominantly static in many companies. Processes are recorded in detail in organizational manuals. When it comes to corporate data, on the other hand, real-time rules. Every hour, thousands of data records and thus information are created or changed in the system, creating new potential knowledge.
In addition to deciding which processes and data are included in the AI knowledge, it must also be ensured conceptually and technically that only the processes and data permitted for them are used by the AI in the interaction with users. The AI must know the role in which it is allowed to act and adhere to its limits. In the above example with the support chatbot, you would certainly want to prevent a customer from asking the bot (the AI) about orders or master data of other customers or even canceling their customer account.
Furthermore, we have already seen that the creative application of knowledge can be a strength of AI. But how do you prevent too much creativity when, for example, the AI acts in the role of an accountant, is supposed to post depreciation and wants to independently determine the depreciation period of the asset? And how can the non-deterministic decisions and results of the AI be tracked later - keyword compliance and auditability? In this context, reference should be made to an interaction with ChatGPT in which the AI allowed itself to be convinced in the course of the conversation that 3 + 4 = 8.
In C-level management, the topic of AI has appeared on the radar at the latest with the hype surrounding ChatGPT. However, the example of blockchain has shown that the mere existence of a new technology without meaningful applications in the corporate context is doomed to failure. In the case of AI, too, it will come down to successful lighthouse projects. As soon as these demonstrate real added value, a large-scale breakthrough of this promising technology is quite possible.