Data Concept for Simplified Data Migration to SAP S/4 Hana
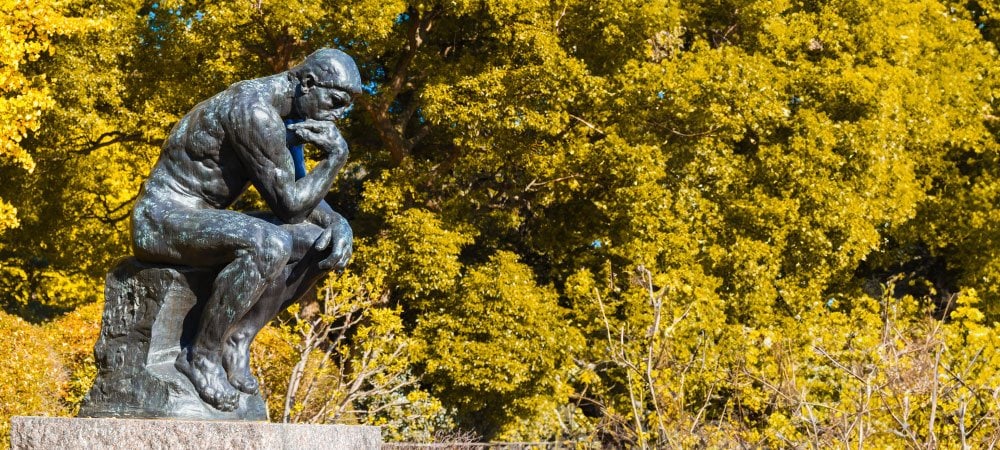
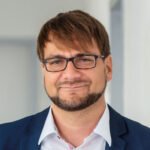
A lack of overview of the data and poor data quality make things even more difficult. A preparatory data concept can make a significant contribution to simplifying data migration for a faster time-to-value. The clock is ticking relentlessly. By 2030 at the latest, companies that want to do so must have migrated to SAP S/4. And such a migration is not so trivial. This is because replacing legacy systems and switching to S/4 involves many different steps, some of which require external support. And external resources are scarce, especially now that the deadline is approaching.
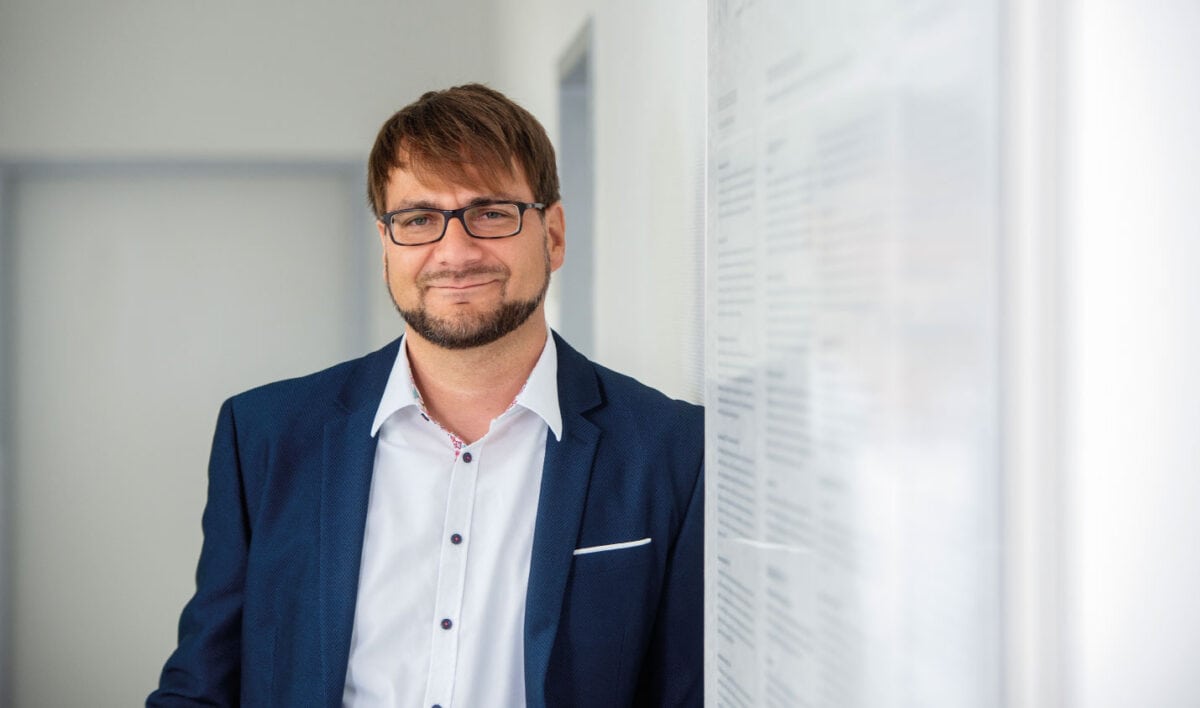
Regardless of which migration approach affected companies choose, whether greenfield, brownfield or bluefield, there are many things that affected companies need to question. Which departments are involved? Who is the main driver and responsible for the migration? Where do I need support, including external support? Which processes are affected? And, and, and.
There are also a lot of things to consider when it comes to data. The new S/4 Hana data model sets the standard. Ideally, migrating companies should reorganize their data. What was previously run separately as a customer, vendor and other business partner must in future be consolidated into the central business partner master in the new data model. As this step will be necessary sooner or later, it is advisable to take data consolidation into account during migration. This is often made more difficult by poor data quality, data storage in silos that has usually grown historically and often extensive customizing.
This almost toxic mix of stressful factors makes data migration a thoroughly challenging sub-project. According to the study 'SAP S/4 Hana 2024 - Current Cloud ERP Trends' by CIO, CSO and Computerwoche, around 39% of respondents see the effort involved in data migration as the biggest challenge when switching to S/4. This was followed in second and third place by the effort required to adapt the existing system landscape and IT architecture (around 37 percent) and a lack of resources for the transformation in the relevant specialist departments (36 percent).
In order to simplify data migration as much as possible, it is first and foremost necessary to address the topic of data as early as possible in the migration project, and not only when the entire migration is as good as complete. In particular, the task is to consolidate existing data from the legacy system(s) (such as ERP/ECC 6.0) to the new S/4 Hana data model, the central business partner master. The key is to simultaneously cleanse and prepare the data in accordance with current data quality standards, in particular postal validation and duplicate cleansing (identity resolution).
A preparatory data concept is an important first step for efficient consolidation to the central business partner master. The data concept bundles all sub-steps that serve to record, describe and finally document the initial situation and the target situation with regard to the data of customers, vendors and other business partners with their roles. In this context, this step also provides a clear overview of the status of the data, the current processes and the degree of customizing that characterizes the current situation. At the same time, the way in which the data is transferred from the old to the new system is determined. But how does such a data concept actually work?
Data exploration, i.e. the very specific examination and investigation of the data, marks the start of the data concept. Without clarity about the actual nature of the data from the various data sources to be migrated, no sensible data concept can be developed.
On the one hand, data exploration looks at the data quality situation. Is the data postally correct? How high is the proportion of duplicate and multiple data records? However, the focus is also on the structural nature of the data in particular. How are the data records structured in principle? Which fields are present? Are these fields actually filled? And are they filled with the correct data? In other words, does a house number field actually contain a house number and does this house number really exist? Or is there a zip code field? And if so, how full is it? And does it always contain plausible data, such as a five-digit zip code in Germany?
It is advisable to load the data to be understood into a data hub such as Uniserv's Customer Data Hub. When working with the data in the data hub, experience has shown that four to five iterations are often necessary until you have really captured and understood your data one hundred percent and, in the end, have truly transparent documentation of what data you actually have.
Actual versus target
The data concept takes up the results of the data exploration and completes the picture of the current situation. In particular, the business processes that are currently being used are recorded. It is also important to understand which departments are involved and where which data is required. A sales department, for example, is interested in sales figures, purchase histories and credit ratings. A finance department, on the other hand, needs payment data and the relevant contact persons.
These different views of one and the same data set and the different data requirements make it clear how complex it is to record and describe the current situation, but also how important it is.
Once you have a complete, transparent and clearly documented view of the current situation, you can start working on the design of the target situation, the goal. The interesting thing here is that you have to describe something that does not yet exist. It is therefore necessary to take a very close look and answer a wide range of questions with regard to the new data model. This includes, for example, determining what the future business processes should look like. Which data flows already exist? Which data flows need to be replaced and which need to be newly created? It must be determined which data is actually needed where and where this data comes from. It is also important to get an overview of where and how interfaces between systems or departments exist.
At this point at the latest, you will be glad if you have worked precisely in the previous steps. Because then, for example, it is also possible to determine whether the existing data quality is sufficient, which data is still needed at all or whether completely new data will be required in the future. This allows you to determine, initially at a theoretical level, which data can be deleted before migration and what data may need to be archived. Which data is required for which processes and applications? This significantly reduces the amount of data to be migrated. Which makes things easier.
Think big, start small
Many companies, especially large corporations, often have an enormous amount of peripheral systems and data in their organizational units and subsidiaries. Anyone who tries to capture everything completely at the first attempt will probably fail, despite a well-planned approach. This is because migrations can often only be carried out step by step; you cannot afford to paralyze your own operations for days on end.
It is therefore advisable to get a big picture in data exploration and data conception. During implementation, however, it is then helpful to work through source by source or system by system. This is because the big picture is created with a view to the overall migration in relation to the new data model. In the continuous processing of sources and systems, these can be migrated in line with the new data model. Positive effect: Lessons learned from each sub-step can flow back into the big picture and sharpen it further.
Solution Design
Once the data concept is in place, you can think specifically about how to get from your current state to the target state. So what should the transition look like? The solution is to build towards the central data point. Is the current solution good as it is, or is something else additional needed?
The solution design therefore answers the question of how the data migration should be implemented in concrete terms. The solution design relates to the overall solution and strategic planning. The existing solution is checked against an alternative solution design. The selection and implementation of the integration architecture and data stewarding interface are addressed.