Document recognition and AI - unspectacular or impressive?
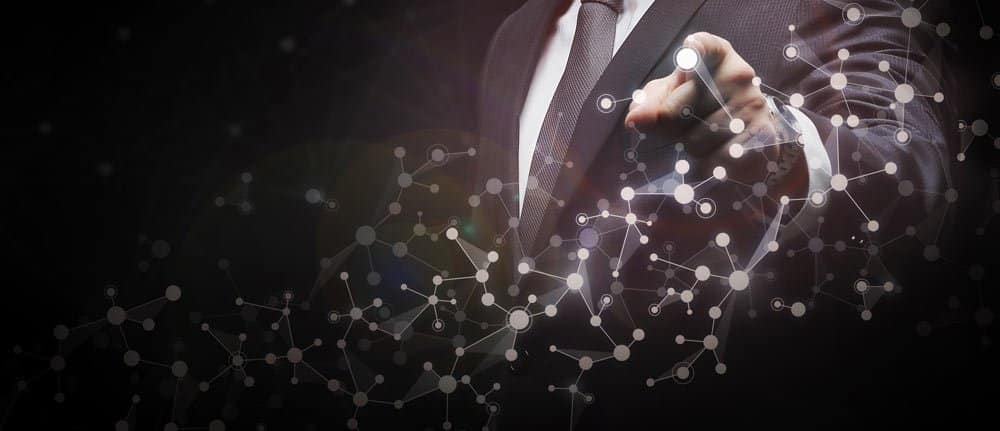
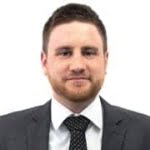
Reading and using information and data from documents is no great challenge for us as humans. We are effortlessly able to sort and separate a stack of the most diverse documents based on their layout and capture all the necessary information.
For software solutions, this processing is anything but mundane. Efficiently extracting information from incoming business documents, such as purchase orders, is critical for companies that deal with countless documents every day.
Especially because scanning and document capture are worlds apart. When a document is scanned, it is stored digitally on the computer. That's where the process stops.
The file is digitized, but users can't do much with the information contained in the document. However, this information is very valuable and companies need it for use and further processing in their SAP system.
Therefore, it is essential to establish a software or process for content document capture. But this is far from the end of the task.
Despite ever-improving technologies, comprehensive semantic correctness of data extraction is still a challenge, especially when analyzing table contents to identify ordered or invoiced items, as the documents often have complex and ambiguous structures.
One can rely on recognition methods similar to face recognition. In combination with a large number of layout templates and continuous machine learning, high automation rates can be generated for the recognition and capture of documents such as orders or invoices.
This method can be supplemented with an intelligent extraction of table contents that goes beyond the mere recognition of physical structures.
This is an approach based on Deep Learning that enables the recognition of positions in different layouts that are not necessarily taken into account in pure structure recognition or are not taught to the algorithm in advance.
The new approach, based on Deep Learning, trains the algorithm used with a large amount of processed real data, which is anonymized for data protection reasons and made available to a neural network.
This algorithm is now able to generate high capture rates even for the initial capture of orders or invoices due to the "experience" and the correspondingly large network.
It is possible to recognize complex table contents in addition to text and numbers for initial orders. Deep Learning as a subset of artificial intelligence helps to significantly increase productivity and operational efficiency.
The novel recognition approach is of particular interest because its analysis logic is generic in principle and can thus be easily adapted to other document types. It is based only to a small extent on specific layout-based text processing.
These technologies show how exceptionally efficient artificial intelligence can be. Work is currently underway on the next generation of AI services that will soon be able to extract accurate and reliable data from purchase orders, invoices and other business documents at the drop of a hat.
What is particularly exciting is that the best AI approaches are exceptionally well suited for natural language processing in the business document domain and represent tremendous potential for innovation in the future.
As unspectacular as document capture may seem to us as humans, the processes behind automated processing not only impressively demonstrate the hurdles, but also the rapid pace of technical development and fascinating approaches to solutions - an impressive challenge to master.