Enterprise AI Chatbots With Open Source Software
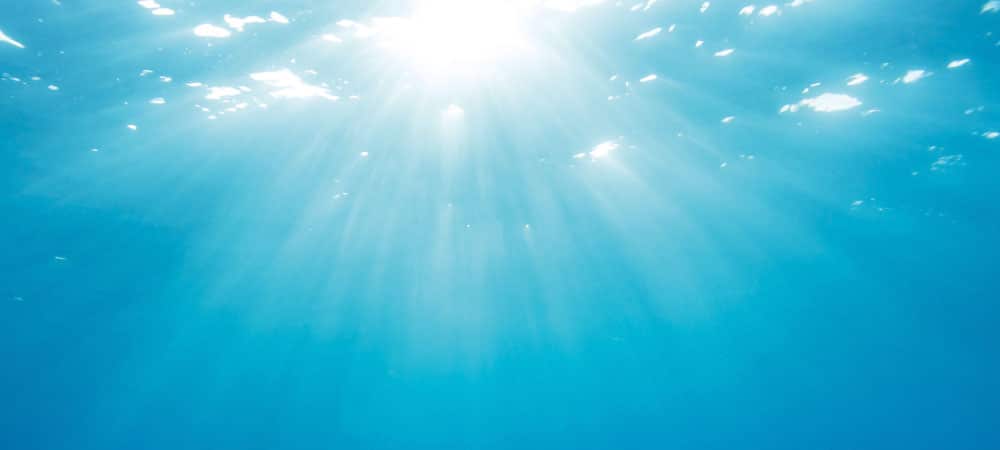
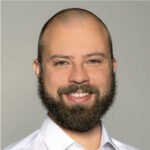
The danger of closed source AI is that, to date, it has not been made public how the language models of major AI chat hosts were trained. On the contrary, there are repeated reports that parts of the training data are copyrighted. As long as the training process is not disclosed, there is always the risk that every message, every website, every text, and thus potentially also internal company data, will be used for training. One thing is also clear: language model developers live off of data and must constantly train language models. Where do they get new data if the entire free internet has already been used?
The groundbreaking feature of large AI chat hosts is not that there is some kind of super intelligence working in the background that can replace humans. Since it is only a matter of calculating probabilities, a chatbot is not an intelligent being. Even though the whole construct is highly complex, the element that promises success is simple: language. Large language models make it possible to interact with computer systems using natural, human language. The interface is simplified even further, like a chat. You Communicate with a computer as if you were writing to a friend on Messenger.
LLM and own data
The support options are huge. A training plan for a successful triathlon? No problem. Want to boil down excruciatingly long emails or contracts to their essentials? Done in seconds. Analyzing anomalies in large SAP log files? Instant results. When you combine an LLM with your own data, the possibilities are almost endless. Imagine what is possible when you can query your entire ticket system as a knowledge base using natural language. Your data is your strength.
LLMs make the most of that strength. LLMs bring your data to life. However, because OpenAI, for example, provides no insight, you can never be certain that your data is safe behind closed doors. But that closed door opens another door: open source.
Open source provides the key. Every detail in the program code can be traced, every adjustment can be checked. That means you know exactly what happens to your data: nothing. Because you're not sending data to a company, you're bringing the product into your own home. It's as if you could buy ChatGPT as a finished product and put it in your own data center. You have the key and full control over if and how your data is connected to AI. In short, the advantage of open source is transparency.
Inference Engine
The first step is installing an inference engine powerful hardware, which enables the operation of language models. The only thing missing is the corresponding LLM, which can be found on Hugging Face for each application. Then you have two options to access your data: fine-tuning and RAG (Retrieval Augmented Generation). While fine-tuning requires too much performance and cost, RAG offers an inexpensive alternative.
RAG does not modify the LLM's data, but provides context from your own database. As if you were saying to the LLM: “Here is my ticket 1234, summarize the content for me.” The fact that the context is always provided has another advantage; unlike online providers, the data set is always up-to-date.
With RAG and an open source LLM, you have everything you need to connect your own data to the technology. The answer to the question of how to keep up with technological change without worrying about the security of your own data is simple: open source.
To the partner entry:
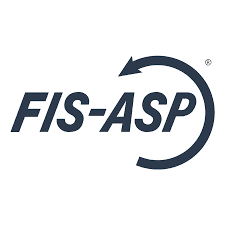