The four musketeers of data quality
![[shutterstock: 555120925, stefano cellai]](https://e3magpmp.greatsolution.dev/wp-content/uploads/2017/01/Musket.jpg)
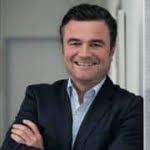
Athos, Porthos, Aramis and D'Artagnan - these are the names of the four musketeers in literature and film. In terms of data quality, their names are data analysis, data cleansing, data protection and data monitoring.
Data analysis: status quo of data quality
Before companies can even begin to look at increasing the amount of customer data available in the enterprise, they must first get an overview of the current state of their data.
For many companies, this first step already represents a major challenge, because the data to be analyzed is usually located in different systems distributed throughout the company.
The most important task in the field of data analysis is to make reliable statements about the nature and quality of customer data, even if it involves a large volume of data.
For example, it is a question of whether the correct information, for example the postal code, is also in the field provided for it. Or to what extent the individual data fields are filled in at all. And whether the data contained is also plausible.
In addition, it is necessary to define company-specific rules and metrics with which the existing data sets can be enriched.
By using appropriate filters and segmentations, the data analysis also enables the detection of "outliers" or "conspicuous features", which can then be dealt with as part of the further quality improvement measures.
Data cleansing: Consolidation of distributed data sets
The second step, data cleansing, is then about correcting the deficiencies and shortcomings in quality previously identified in the analysis.
For this purpose, the existing data is extracted from the various source systems in the company by using native connectors.
The data records are checked postally, examined for duplicate and multiple data records and, if necessary, can be enriched with additional information such as geo-data or secondary statistical information.
This is where the foundation is laid for the so-called "Golden Record", the "mother of all master records".
Data protection: For sustainable quality assurance
Data protection at this point does not mean the protection of data in the legal sense, but rather measures to ensure that the high quality of customer data previously created can also be maintained and further expanded.
The main focus is on checking the data for errors as soon as possible when it is newly entered or changed.
In this way, hearing, reading and/or typing errors can be noticed, displayed and then also corrected immediately when a data record is created.
Data monitoring: trust is good, control is better
And ultimately, data monitoring ensures that the work of the other three building blocks has not been in vain.
If this does not happen, experience in many companies shows that, in turn, a "creeping contamination" of customer data gradually occurs, which in most cases is unfortunately only recognized when it is too late.
Reasons for this include relocations, divorces, deaths, but also street and town renamings or incorporations.
This meant that the measures implemented in the other three phases and the associated efforts were then also practically in vain.
This is a kind of sensor for data quality weaknesses, so to speak, which ensures that these weaknesses are detected at an early stage before they have an impact on the target system.
The basis for this are the rules and guidelines for data quality defined by the company itself. These guidelines are also reviewed with regard to necessary changes and updates.
Successful optimization of customer data is only possible through an integrated approach. Many companies have now recognized the importance of a high level of data quality as a prerequisite for smooth business processes in a wide variety of areas.
Unfortunately, however, they set different priorities and often focus their efforts only on certain measures. And they forget that the motto of the four musketeers quoted at the beginning of this article also applies to master data management.
What good is a detailed data analysis if the appropriate measures for data cleansing are not derived from it?
And even the positive effects of an initial cleanup will quickly be "diluted" again if no measures are taken to sustainably maintain a high level of data quality.
And ultimately, even the best monitoring in the context of data monitoring is of no use if the results do not flow into a renewed data analysis and thus trigger a renewed process to improve data quality.
This also makes it clear that initiatives to improve the quality of customer data processed within the company are not a temporary process or even a one-off action.
Instead, an integrated and continuous closed loop is required to achieve sustainable optimization and assurance of data quality: One for all, all for one.
The goal: 360-degree view of the customer
While there are certainly one-time events such as the installation of a new CRM system, an ERP migration, or even a company acquisition that require migration and consolidation of data, and thus can be the trigger for a data quality optimization initiative, there are also times when the data quality of a company has to be optimized.
However, in most cases today, when companies look at optimizing the quality of their customer data, they are concerned with obtaining the most accurate, complete, and up-to-date 360-degree view of the customer possible.
So that they can optimally accompany their customers through the individual phases of the customer journey.
Ultimately, the 360-degree view of the customer plays the central role in retail when it comes to positioning oneself as an attractive companion to the customer and thus binding him to the company in the medium term.
In times of multi-, omni- and cross-channel sales, the aim is to serve all customer touch points - offline and digital - with information and offers that are individually tailored to the customer and authentic.
From Golden Record to Golden Profile
The more digital the customer becomes, the more important it is for companies to not only capture and consolidate the data and information known about a customer within the company, but also to track the "traces" that the customer leaves behind on the Internet and social networks today.
With Ground Truth, Uniserv has therefore developed a solution and process methodology which supports companies accordingly in ultimately creating the Golden Profile of each customer in a multi-stage procedure which aggregates their address data, their purchasing behavior, their interests and preferences, but also their communication and interaction with the company into a central data record.
In addition, Golden Profiles integrate the "traces" that the customer leaves behind on the Internet and social networks.
In other words, the master data of each customer (golden record) and the transaction data (transaction and interaction data) are merged (golden profile). Ground Truth also ensures that this data is continuously updated and synchronized across the various sources.
Uniserv developed a prototype based on ground truth especially for predictive analytics in cooperation with Stuttgart Media University.
This prototype was used to illustrate the importance of data quality as a critical success factor for the quality of forecasts.
Conclusion
One for all, all for one: This guiding principle not only applied to the four musketeers in literature and film, this guiding principle also and especially applies to the four musketeers in data quality: data analysis, data enhancement, data protection and data monitoring.
Each building block in itself requires careful planning and implementation, but only in smooth interaction and integration to form a closed loop does the data quality in the company reach a completely new level that can be maintained sustainably and successively optimized.
Only then is the basis created for the use of ground truth. And only then does the company ultimately achieve a precise, complete, and up-to-date 360-degree view of the customer and thus a basic confidence in its own data quality.