Gödel, Escher, Bach: an Eternal Golden Braid
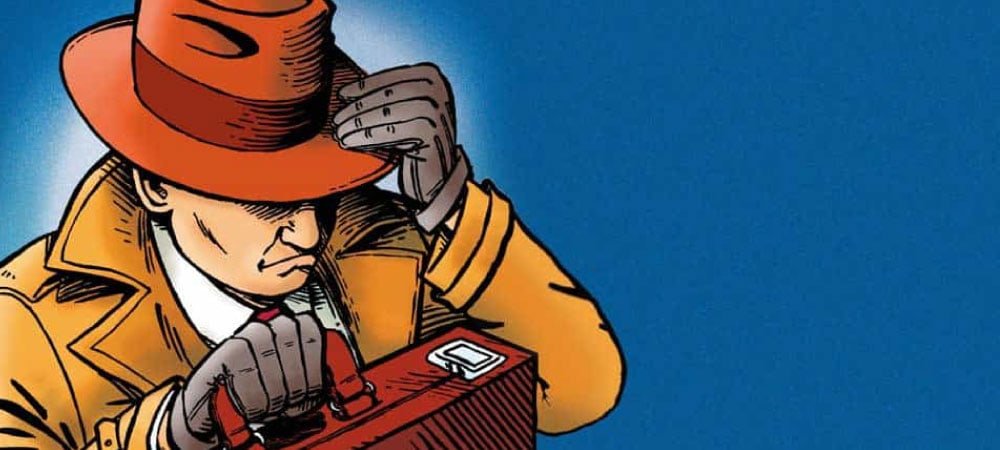
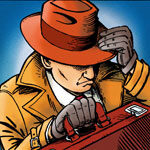
A few years ago, I attended an AI conference organized by the German newspaper Handelsblatt in Munich, and was able to behold computer scientist and AI pioneer Jürgen Schmidhuber during his lecture on deep learning, recurrent neural networks, and long/short term memory. At the time, machine learning was a dominant topic due to Google's DeepMind and its AlphaGo program.
Jürgen Schmidhuber’s comment motivated me to purchase and read the book "Gödel, Escher, Bach: an Eternal Golden Braid" by Douglas R. Hofstadter. Schmidhuber said that DeepMind has not only built a neural network that plays Go better than any human, but that neural networks will soon build even better neural networks—in other words, a back-referenced AI.
The thought makes my head spin. And why is that? Because there is a paradox in many AI developments, called the barber paradox. The barber is "one who shaves all those, and those only, who do not shave themselves." Does the barber then shave himself? This is a paradox because the barber cannot shave himself, if he only shaves those who do not shave themselves. With this paradox in mind, it's not surprising that generative AI begins to hallucinate.
Douglas R. Hofstadter's book does not provide an answer to paradoxes and fantasies. What it does do is provide a generally understandable introduction to the world of formal systems. It introduced me to Gödel's incompleteness theorem, which states that every complex system contains statements that can neither be proven nor disproven by means of the system. As the Austrian Kurt Gödel showed, contradiction-free formal systems reach their limits when they make statements about themselves, see the barber paradox or "this sentence is not true" (liar's paradox).
Against this amusing and theoretical backdrop, I wonder—as an SAP customer— whether we will ever be able to use generative AI as an ERP bot. An AI system in a financial system environment should probably not hallucinate. For trivial tasks, such as providing up-to-date financial analyses based on Excel and statistics, the cost of a generative system is too high for me. SAP already provides us with AI modules and functions, but their intelligence is quite modest.
SAP itself has not yet ventured into the area of large-language models, which is the basis for generative AI. However, there are official interfaces to connect with OpenAI via the SAP Business Technology Platform, and SAP is supporting Jonas Andrulis' startup Aleph Alpha with several million euros.
SAP executive board members Jürgen Müller and Thomas Saueressig have not yet presented us with an ERP AI master plan that would include an S/4 AI roadmap through to 2040. Individual pieces of the puzzle for improved, generative AI, such as a Hana Cloud Vector Engine, are probably the right way to go, but they not enough. The SAP Vector Engine should combine the power of LLM (large language models) with relevant data from the ERP to ensure that business-relevant information and context is maintained in the generative AI’s results.
Jonas Andrulis also recommended the combination of process mining and large language models at a recent Handelsblatt conference. He stated he could imagine combining business process insights based on Signavio's process mining with Aleph Alpha's LLM. If SAP's Chief Technology Officer Jürgen Müller were to contribute his Vector Engine as Graph Computing, such a system could not only provide relevant business insights, but perhaps also be hardened against AI hallucinations.
SAP remains silent and, in my personal opinion, is approaching the topic of AI far too hesitantly and cautiously. Is SAP missing out on the AI trend?
Of course, it takes courage to embark on this journey, which can also end in an eternal staircase to nowhere, like in one the Dutch draftsman and graphic artist Maurits Cornelis Escher's famous pictures. He depicted optical illusions, logical contradictions, and strange loops like no other. The aforementioned image of men walking up an endless staircase without actually gaining or losing distance is well known.